Catalogue Search | MBRL
نتائج البحث
MBRLSearchResults
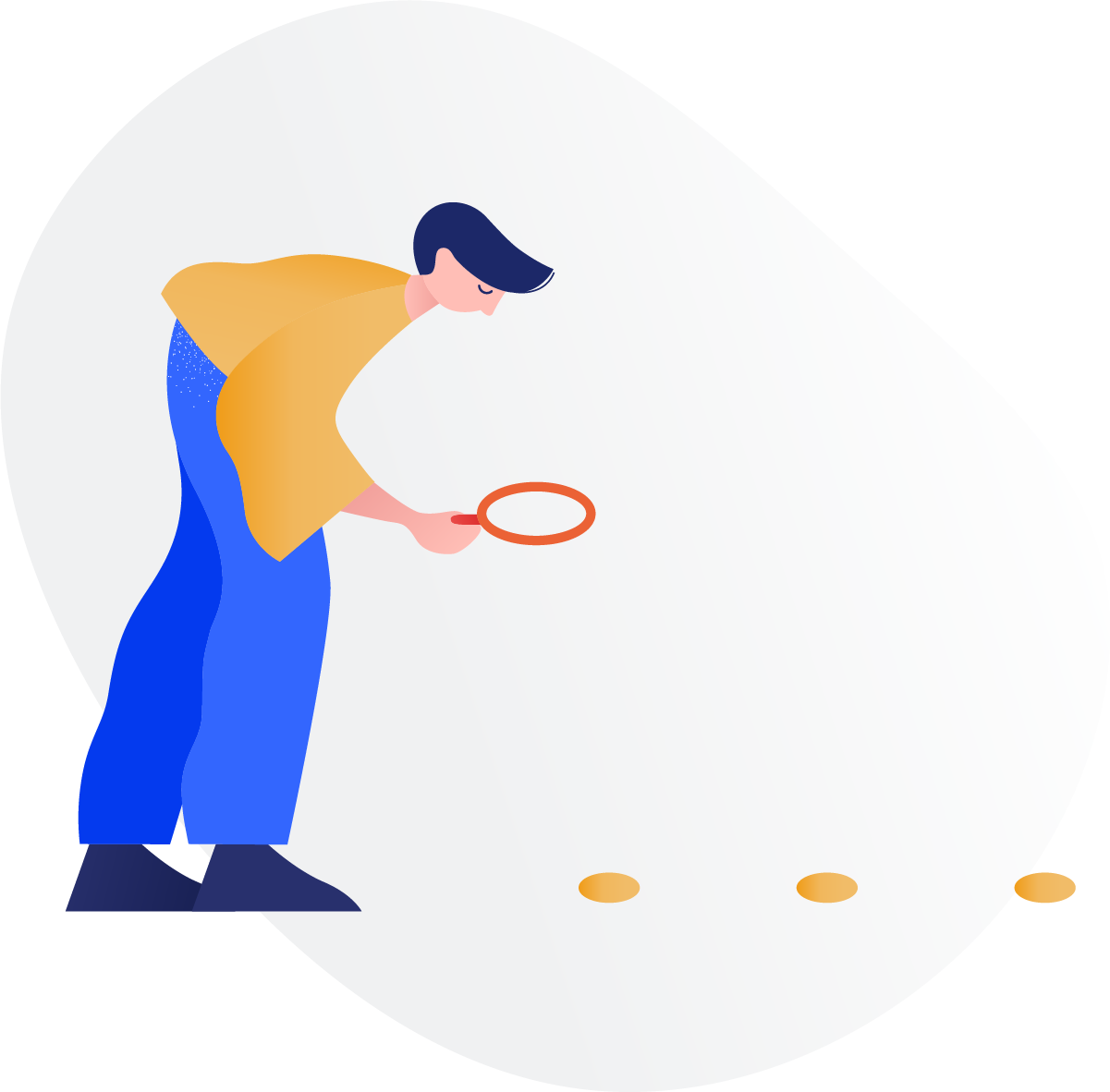
وجه الفتاة! هناك خطأ ما.
أثناء محاولة إضافة العنوان إلى الرف ، حدث خطأ ما :( يرجى إعادة المحاولة لاحقًا!
-
الضبطالضبط
-
مُحَكَّمةمُحَكَّمة
-
نوع العنصرنوع العنصر
-
الموضوعالموضوع
-
السنةمن:-إلى:
-
المزيد من المرشحاتالمزيد من المرشحاتالمصدراللغة
منجز
مرشحات
إعادة تعيين
219
نتائج ل
"Sharma, Alok Kumar"
صنف حسب:
The Role of Ultrasound in Cancer and Cancer-Related Pain—A Bibliometric Analysis and Future Perspectives
بواسطة
Lim, Hae Gyun
,
Sharma, Alok Kumar
,
Sridharan, Badrinathan
في
acousitc waves
,
bibliometry
,
Cancer
2023
Ultrasound has a deep penetrating ability with minimal or no tissue injury, while cancer-mediated complications during diagnosis, therapy, and surgery have become a serious challenge for clinicians and lead to the severity of the primary condition (cancer). The current study highlights the importance of ultrasound imaging and focused ultrasound therapy during cancer diagnosis, pain reduction, guidance for surgical resection of cancer, and the effectiveness of chemotherapy. We performed the bibliometric analysis on research domains involving ultrasound, cancer management, pain, and other challenges (chemotherapy, surgical guidance, and postoperative care), to observe the trend by which the research field has grown over the years and propose a possible future trend. The data was obtained from the Web of Science, processed, and exported as plain text files for analysis in the Bibliometrix R web interface using the Biblioshiny package. A total of 3248 documents were identified from 1100 journal sources. A total of 390 articles were published in 2022, with almost a 100% growth rate from previous years. Based on the various network analysis, we conclude that the outcome of the constant research in this domain will result in better patient care during the management of various diseases, including cancer and other co-morbidities.
Journal Article
Estimation of Gait Parameters for Adults with Surface Electromyogram Based on Machine Learning Models
بواسطة
Sharma, Alok Kumar
,
Wang, Jia-Jung
,
Ting, Chi-En
في
Adult
,
Biomechanical Phenomena
,
Biomechanics
2024
Gait analysis has been studied over the last few decades as the best way to objectively assess the technical outcome of a procedure designed to improve gait. The treating physician can understand the type of gait problem, gain insight into the etiology, and find the best treatment with gait analysis. The gait parameters are the kinematics, including the temporal and spatial parameters, and lack the activity information of skeletal muscles. Thus, the gait analysis measures not only the three-dimensional temporal and spatial graphs of kinematics but also the surface electromyograms (sEMGs) of the lower limbs. Now, the shoe-worn GaitUp Physilog
wearable inertial sensors can easily measure the gait parameters when subjects are walking on the general ground. However, it cannot measure muscle activity. The aim of this study is to measure the gait parameters using the sEMGs of the lower limbs. A self-made wireless device was used to measure the sEMGs from the vastus lateralis and gastrocnemius muscles of the left and right feet. Twenty young female subjects with a skeletal muscle index (SMI) below 5.7 kg/m
were recruited for this study and examined by the InBody 270 instrument. Four parameters of sEMG were used to estimate 23 gait parameters. They were measured using the GaitUp Physilog
wearable inertial sensors with three machine learning models, including random forest (RF), decision tree (DT), and XGBoost. The results show that 14 gait parameters could be well-estimated, and their correlation coefficients are above 0.800. This study signifies a step towards a more comprehensive analysis of gait with only sEMGs.
Journal Article
Human Activity Recognition Based on Deep Learning and Micro-Doppler Radar Data
بواسطة
Sharma, Alok Kumar
,
Huang, Yung-Fa
,
Tian, Jia-Hong
في
Algorithms
,
cross-channel operation
,
Deep Learning
2024
Activity recognition is one of the significant technologies accompanying the development of the Internet of Things (IoT). It can help in recording daily life activities or reporting emergencies, thus improving the user's quality of life and safety, and even easing the workload of caregivers. This study proposes a human activity recognition (HAR) system based on activity data obtained via the micro-Doppler effect, combining a two-stream one-dimensional convolutional neural network (1D-CNN) with a bidirectional gated recurrent unit (BiGRU). Initially, radar sensor data are used to generate information related to time and frequency responses using short-time Fourier transform (STFT). Subsequently, the magnitudes and phase values are calculated and fed into the 1D-CNN and Bi-GRU models to extract spatial and temporal features for subsequent model training and activity recognition. Additionally, we propose a simple cross-channel operation (CCO) to facilitate the exchange of magnitude and phase features between parallel convolutional layers. An open dataset collected through radar, named Rad-HAR, is employed for model training and performance evaluation. Experimental results demonstrate that the proposed 1D-CNN+CCO-BiGRU model demonstrated superior performance, achieving an impressive accuracy rate of 98.2%. This outperformance of existing systems with the radar sensor underscores the proposed model's potential applicability in real-world scenarios, marking a significant advancement in the field of HAR within the IoT framework.
Journal Article
STB: synthetic minority oversampling technique for tree-boosting models for imbalanced datasets of intrusion detection systems
بواسطة
Sharma, Alok Kumar
,
Li, Li-Hua
,
Ahmad, Ramli
في
CatBoost
,
Intrusion detection system
,
LightGBM
2023
Attacks on the Intrusion Detection System (IDS) can result in an imbalanced dataset, making it difficult to predict what types of attacks will occur. A novel method called SMOTE Tree Boosting (STB) is proposed to generate synthetic tabular data from imbalanced datasets using the Synthetic Minority Oversampling Technique (SMOTE) method. In this experiment, multiple datasets were used along with three boosting-based machine learning algorithms (LightGBM, XGBoost, and CatBoost). Our results show that using SMOTE improves the content accuracy of the LightGBM and XGBoost algorithms. Using SMOTE also helps to better predict computational processes. proven by its accuracy and F1 score, which average 99%, which is higher than several previous studies attempting to solve the same problem known as imbalanced IDS datasets. Based on an analysis of the three IDS datasets, the average computation time required for the LightGBM model is 2.29 seconds, 11.58 seconds for the XGBoost model, and 52.9 seconds for the CatBoost model. This shows that our proposed model is able to process data quickly.
Journal Article
A Novel Fuzzy DBNet for Medical Image Segmentation
بواسطة
Huang, Pei-Chen
,
Chang, Sheng-Wen
,
Chin, Chiun-Li
في
Algorithms
,
anteroposterior
,
butterfly network
2023
When doctors are fatigued, they often make diagnostic errors. Similarly, pharmacists may also make mistakes in dispensing medication. Therefore, object segmentation plays a vital role in many healthcare-related areas, such as symptom analysis in biomedical imaging and drug classification. However, many traditional deep-learning algorithms use a single view of an image for segmentation or classification. When the image is blurry or incomplete, these algorithms fail to segment the pathological area or the shape of the drugs accurately, which can then affect subsequent treatment plans. Consequently, we propose the Fuzzy DBNet, which combines the dual butterfly network and the fuzzy ASPP in a deep-learning network and processes images from both sides of an object simultaneously. Our experiments used multi-category pill and lung X-ray datasets for training. The average Dice coefficient of our proposed model reached 95.05% in multi-pill segmentation and 97.05% in lung segmentation. The results showed that our proposed model outperformed other state-of-the-art networks in both applications, demonstrating that our model can use multiple views of an image to obtain image segmentation or identification.
Journal Article
The role of stress personalities: a comparative study between Indian Academia and industry
بواسطة
Kumar, Vimal
,
Sharma, Alok Kumar
,
Mittal, Ankesh
في
Academic staff
,
Comparative studies
,
Consultants
2022
Purpose
Stress is the most common emotional or mental state that employees experience during their work. The employees in academics and industry are facing increased levels of stress as they progress through their work. The study aims to investigate the relationship between academic and industry employees’ stress personalities. West Coast psychological consultants Mary Dempcy and Rene Tihista distinguish between the seven various types of stress and offer suggestions to deal with it.
Design/methodology/approach
In this study, the authors have built a survey questionnaire using a sample of 195 respondents from the industry and academic of North India and analysed their responses to find their stress personalities at work. The Independent sample t-test approach has been applied to analyse the employee stress personality.
Findings
The study finds out that stress is a sophisticated defence mechanism that is unique to each individual and varies depending on the environment. Using employee response of academic and industry, the study covers the essence of seven types of stress for individuals, and that lent good support to the framed hypothesis.
Research limitations/implications
These seven types of stress have importance and different levels to knowing their appropriateness to the individuals and suggest to take necessary action of plan. It shows the individuals feel about stress, how the bodies react to it and how to cope with it are all indicators of the personalities, attitudes and adaptability.
Originality/value
The novelty of this study is to apply Mary Dempcy and Rene Tihista’s stress personalities compared with the two respective categories.
Journal Article
A Study on Recommender Systems
بواسطة
Agrima, Mehandiratta
,
Sharma, Alok Kumar
,
Pooja, Gupta
في
collaborative recommender
,
content‐based recommender system
,
e‐commerce
2021
In today's world, where we have an abundance of data collected over the years, Machine Learning aims to put this data to use. This data can be used to train machines to accurately predict the behavioral patterns of users, thus making many processes automated. This project explores one such specimen being implemented in the field of e‐commerce today: Recommender system. As shopping moves from the physical to the online world, providing products to customers according to personal preferences becomes a bigger challenge. Recommender systems are the solution to this problem. Most recommender systems in practice today use complex characteristics to improve performance and results. However, in this exposition, we explored a simpler approach to recommender systems that are fairly easy to use for small scale e‐retail websites. Further, we tried and tested small variations in the data set and compared their performances, therefore, obtaining the best performing algorithm.
Book Chapter
Lepton flavor non-universality in the B-sector: a global analyses of various new physics models
بواسطة
Kumar, Dinesh
,
Kumar, Jacky
,
Sharma, Ruchi
في
Astronomy
,
Astrophysics and Cosmology
,
Elementary Particles
2019
The measurements of the ratios
R
K
(
∗
)
along with
R
D
(
∗
)
hint towards lepton flavor non universality which is in disagreement with the standard model. In this work, we reanalyze the four new physics models, which are widely studied in the literature as a candidates for the simultaneous explanations of these measurements. These are, standard model like vector boson (VB),
S
U
(
2
)
L
-singlet vector leptoquark (
U
1
),
S
U
(
2
)
L
-triplet scalar leptoquark (
S
3
) and
S
U
(
2
)
L
triplet vector leptoquark (
U
3
) models. We assume a coupling only to the third generation in the weak basis, so that the
b
→
s
μ
+
μ
-
transition is generated only via mixing effects. Preforming a global fit to all relevant data, we show that the vector boson model violates the current upper bound on
Br
(
τ
→
3
μ
)
and hence is
inconsistent
with the present data. Further, we show that within this framework, the
U
1
leptoquark model
cannot
simultaneously accommodate
R
K
(
∗
)
and
R
D
(
∗
)
measurements. We emphasize that this conclusion is independent of the additional constraints coming from renormaliztion group running effects and high-
p
T
searches. In addition, we show that the
S
3
and
U
3
models are highly disfavored by the constraints coming from
b
→
s
ν
ν
¯
data. Finally, we find a that hypothesis of two LQ particles is also challenged by
b
→
s
ν
¯
ν
data.
Journal Article
An improved discriminative filter bank selection approach for motor imagery EEG signal classification using mutual information
بواسطة
Kumar, Shiu
,
Tsunoda, Tatsuhiko
,
Sharma, Alok
في
Banks (Finance)
,
Brain computer interface
,
Common spatial pattern
2017
Common spatial pattern (CSP) has been an effective technique for feature extraction in electroencephalography (EEG) based brain computer interfaces (BCIs). However, motor imagery EEG signal feature extraction using CSP generally depends on the selection of the frequency bands to a great extent.
In this study, we propose a mutual information based frequency band selection approach. The idea of the proposed method is to utilize the information from all the available channels for effectively selecting the most discriminative filter banks. CSP features are extracted from multiple overlapping sub-bands. An additional sub-band has been introduced that cover the wide frequency band (7-30 Hz) and two different types of features are extracted using CSP and common spatio-spectral pattern techniques, respectively. Mutual information is then computed from the extracted features of each of these bands and the top filter banks are selected for further processing. Linear discriminant analysis is applied to the features extracted from each of the filter banks. The scores are fused together, and classification is done using support vector machine.
The proposed method is evaluated using BCI Competition III dataset IVa, BCI Competition IV dataset I and BCI Competition IV dataset IIb, and it outperformed all other competing methods achieving the lowest misclassification rate and the highest kappa coefficient on all three datasets.
Introducing a wide sub-band and using mutual information for selecting the most discriminative sub-bands, the proposed method shows improvement in motor imagery EEG signal classification.
Journal Article